I very occasionally visit Xitter (pronounciation tip: it goes like the name of the President of the People’s Republic of China). The other day, Jason Huggins said
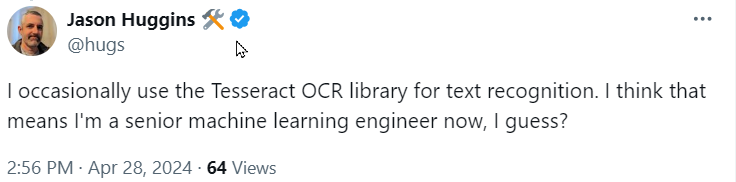
Just in case you’re using a screen reader, that’s “I occasionally use the Tesseract OCR library for text recognition. I think that means I’m a senior machine learning engineer now, I guess.”
I felt a little impish, but I also felt quite lazy. This seemed like a perfect application for ChatGPT.
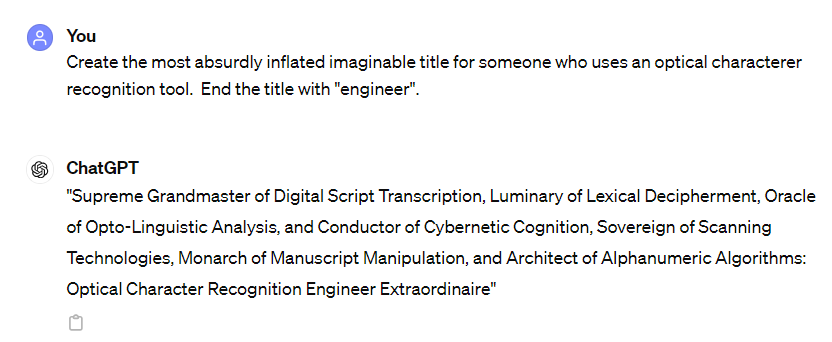
I prompted: “Create the most absurdly inflated imaginable title for someone who uses an optical characterer recognition tool. End the title with ‘engineer’.”
ChatGPT replied: “Supreme Grandmaster of Digital Script Transcription, Luminary of Lexical Decipherment, Oracle of Opto-Linguistic Analysis, and Conductor of Cybernetic Cognition, Sovereign of Scanning Technologies, Monarch of Manuscript Manipulation, and Architect of Alphanumeric Algorithms: Optical Character Recognition Engineer Extraordinaire”
A few observations: ChatGPT produced a very impressive result. The response was useful for exactly the purpose I had intended: generating lots of words with no particular meaning, with little effort on my part, and with no real regard for truth. That is, bullshit. That sounds like a diss, but in this case it isn’t; often bullshit is pernicious, but sometimes it’s funny, and fun.
But notice something else: ChatGPT got a key part of the assignment wrong. Despite the explicit instruction in the prompt, ChatGPT ended the response with “Extraordinaire”, instead of “Engineer”.
In this case, of course it doesn’t matter. But we should seriously consider what this means for circumstances in which someone might be using an LLM for something that does matter, especially in cases where we would need expert attention to notice and address problems in the output.
I’ve done lots of basic experiments with LLMs that should not pose much of a challenge. Every time, there have been bugs and problems in the responses. The wider literature is full of deeper analysis that points to serious risk. So if you’re using these tools for something important, you’ll need a tester’s mindset to be alert to trouble, a skilled supervisor’s expertise to fix it, and the patience and determination to apply them.
I immediately noticed it got that key part of the assignment wrong too! However, in this very low-stakes, humorous scenario, it was actually a really funny error. It almost (well, you could imagine if you improperly anthropomorphize it as we are apt to do) seems intentional. The “yada yada Engineer… Extraordinaire!” flourish gives it that extra silly punch because it seems to deliberately violate your prompt just slightly.
Of course it isn’t at all, it just messed up for all of the detailed reasons you’ve helped shed light on. Its so frustrating!
It’s all true, but
You know the thing is with testing. If you would have a A team that test sublime… but the net income at the end of the year is 10K.
If you would have a team B that tests less sublime…using a.i. though, but the income at the end of the year is 10Million!
Would it matter that the a.i. makes some mistakes too?
I hear you saying: yes but what if one of that mistakes causes a terrible disaster.
Okay…. so you’re saying humans can not cause that?
So I’m still looking for the #1 argument…
The second part of your reply is based on a logical error that I see the Non-critical AI Fanboys making all the time. It amounts to
Humans are pretty clever, most of the time; and we have to supervise them, because humans make mistakes that might cause terrible disasters, so
LLMs might make mistakes that cause terrible disasters, and we have to supervise them therefore LLMs are pretty clever.
I’m sorry, but the first part of your reply makes no sense. It might even be a good idea to run your idea through a LLM, and see if it can help you to produce something more cogent.
A.i. Fanboys? I consider myself a senior citizen, but okay.
You say:
The second part of your reply is based on a logical error…
later-on you write:
But the first part of your reply makes no sense.
Everything you say is still not a convincing argument(s) to NOT use a.i. in software development (and/or testing).
Please convince me better.
You may have missed the last paragraph and especially the link in the first sentence.
We already have plenty of moderately unreliable software. Using highly unreliable software to test moderately unreliable software doesn’t seem like a winning strategy. People are, of course, free to do what they like, as long as they’re willing to accept the consequences.